In today’s rapidly evolving digital landscape, organizations are increasingly turning to automation technologies to streamline operations, drive efficiency, and stay competitive. One of the most significant developments in the field of automation is the convergence of Robotic Process Automation (RPA) with Artificial Intelligence (AI) and Machine Learning (ML). This convergence represents a paradigm shift in automation, enabling organizations to move beyond repetitive, rule-based tasks towards intelligent automation solutions that can mimic human cognitive functions.
Understanding Intelligent Automation
Intelligent automation combines the efficiency of RPA with the cognitive capabilities of AI and ML, allowing organizations to deploy automation solutions that can adapt, learn, and make data-driven decisions autonomously. By leveraging AI and ML algorithms, intelligent automation can analyze vast amounts of data, recognize patterns, and make predictions, enabling organizations to automate complex tasks that were previously beyond the scope of traditional RPA.
Drivers of Convergence
Several key drivers are fueling the convergence of RPA and AI. Organizations are increasingly recognizing the need for automation solutions that can handle more complex tasks and adapt to changing business requirements. Additionally, advancements in AI and ML technologies, such as natural language processing (NLP) and computer vision, have made it possible to automate tasks that require human-like reasoning and decision-making.
Challenges and Considerations
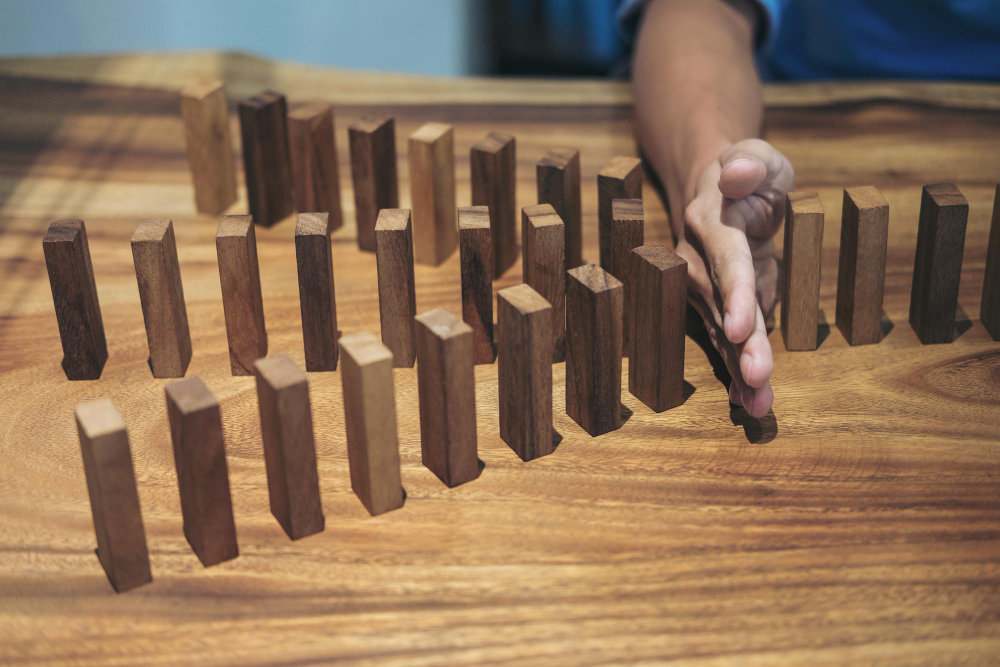
While the convergence of RPA and AI holds tremendous potential, it also presents several challenges and considerations. Organizations must address issues such as data quality, governance, and ethical implications to ensure the responsible use of intelligent automation. Additionally, there may be resistance to change from employees who fear job displacement or lack the necessary skills to work with AI-powered automation tools.
Real-World Use Cases
Real-world use cases of intelligent automation abound across industries. In finance, organizations are using AI-powered chatbots to automate customer support inquiries and improve response times. In healthcare, AI algorithms are analyzing medical imaging data to assist physicians in diagnosing diseases more accurately. In manufacturing, predictive analytics is being used to optimize production schedules and minimize downtime.
Best Practices
Successfully implementing intelligent automation requires collaboration between IT and business stakeholders, continuous learning, and scalability. Organizations should invest in training programs to equip employees with the skills needed to work with AI-powered automation tools effectively. Additionally, they should establish governance frameworks to ensure the responsible use of AI and ML technologies and monitor automation initiatives to measure their impact and identify areas for improvement.
Feel free to share your thoughts and experiences with on-demand computing in the comments below! Let’s get the conversation started.